Pocket-Sized AI Models, AI models, pocket-sized AI, efficient computing, Phi-3-mini, Microsoft AI, local AI processing, AI innovation, AI technology, ChatGPT alternatives, localized AI
Discover how pocket-sized AI models like Microsoft’s Phi-3-mini are revolutionizing computing by offering powerful, efficient AI capabilities on personal devices. Learn about the advancements in AI technology that allow for local processing, enhanced privacy, and new innovative applications.
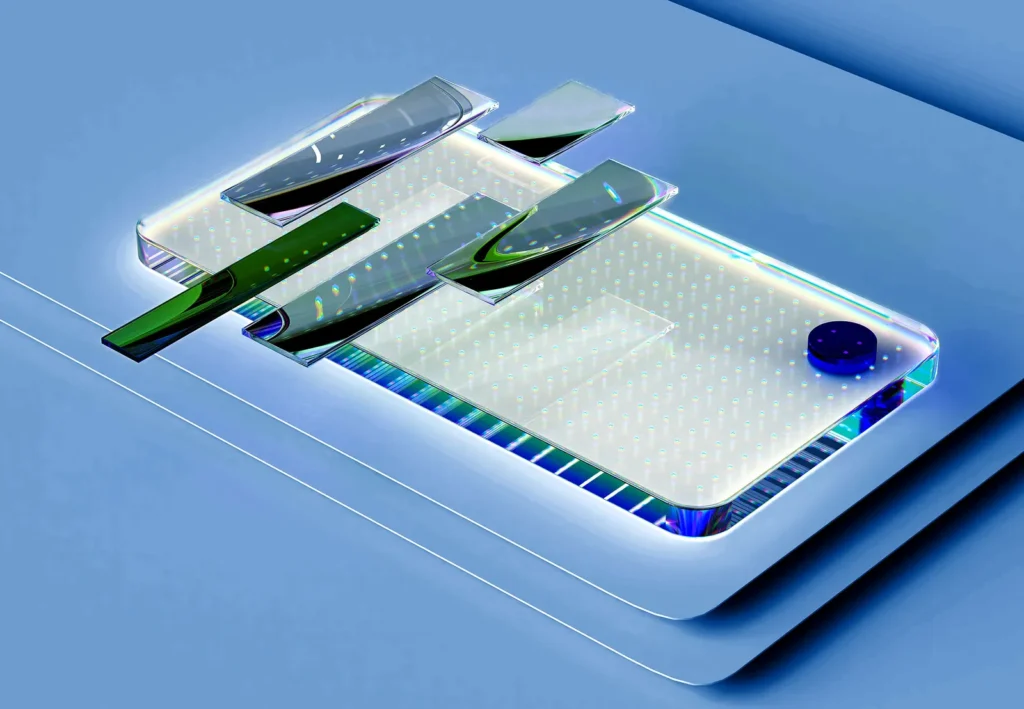
When ChatGPT was released in November 2023, it was accessible only through the cloud due to the immense size of the underlying model. Today, a similarly capable AI program can run on a MacBook Air without even warming up. This rapid refinement of AI models into leaner and more efficient versions demonstrates that scaling up isn’t the only method to enhance machine intelligence.
The AI model powering my laptop, known as Phi-3-mini, is part of a family of smaller models released by Microsoft. While compact enough to run on a smartphone, I tested it on a laptop and accessed it through an iPhone app called Enchanted, which offers a chat interface similar to the official ChatGPT app. Microsoft’s researchers claim in a paper that Phi-3-mini performs comparably to GPT-3.5, the model behind the first release of ChatGPT, based on standard AI benchmarks measuring common sense and reasoning. My testing confirms its impressive capabilities.
At Microsoft’s annual developer conference, Build, they announced a new “multimodal” Phi-3 model capable of handling audio, video, and text. This announcement followed shortly after OpenAI and Google introduced radical new AI assistants built on multimodal models accessed via the cloud. Microsoft’s compact Phi models suggest the possibility of creating various AI applications that don’t rely on cloud connectivity. This shift could enable more responsive and private applications, as offline algorithms can make everything on a PC searchable.
The Phi family’s development also offers insights into modern AI’s nature and potential improvements. Sébastien Bubeck, a researcher at Microsoft, explains that the models were designed to test whether selective training data could fine-tune AI abilities without increasing the model’s size. Large language models like OpenAI’s GPT-4 or Google’s Gemini, which power chatbots and other services, typically use vast amounts of text from various sources. Despite legal questions, feeding these models more text and using more computing power has been shown to unlock new capabilities.
Bubeck, interested in the intelligence of language models, explored whether carefully curated training data could enhance a model’s abilities. In September, his team trained a model one-seventeenth the size of GPT-3.5 on “textbook quality” synthetic data generated by a larger AI model, including factoids from specific domains like programming. The resulting model outperformed GPT-3.5 in coding tasks, demonstrating surprising abilities for its size. This success suggests that targeted training data can make smaller AI models remarkably effective.
Further experiments by Bubeck’s team revealed that even an extra-tiny model trained on children’s stories could produce coherent output, unlike conventionally trained AI of the same size. These findings indicate that future AI systems’ intelligence will depend on more than just scaling up their size. Scaled-down models like Phi-3 could play a significant role in computing’s future, running locally on devices to reduce latency, ensure data privacy, and enable new AI use cases integrated into operating systems.
Apple is expected to unveil its AI strategy at its WWDC conference next month, emphasizing local machine learning on its devices. Rather than competing with OpenAI and Google in developing larger cloud AI models, Apple might focus on shrinking AI to fit into customers’ pockets, leveraging its custom hardware and software for efficient, localized machine learning.
The emergence of pocket-sized AI models like Phi-3-mini marks a transformative shift in computing. These models offer the potential for powerful, efficient AI applications that can operate independently of the cloud, enhancing responsiveness and privacy. By refining AI training methods and focusing on targeted data, researchers are paving the way for smarter, more versatile AI systems that could revolutionize technology and everyday life.
As AI technology continues to evolve, the implications of smaller, more efficient models are vast. They could enable a new wave of innovation in personal devices, making advanced AI capabilities more accessible and integrated into daily tasks. The shift towards localized AI processing also raises exciting possibilities for enhanced privacy and security, as data no longer needs to be transmitted to remote servers for processing.
In conclusion, the development of pocket-sized AI models like Phi-3-mini represents a significant advancement in the field of artificial intelligence. These models not only demonstrate the potential for more efficient and capable AI systems but also highlight the importance of targeted training data and local processing. As companies like Microsoft and Apple continue to explore and expand these capabilities, we can anticipate a future where powerful AI is seamlessly integrated into our personal devices, enhancing functionality, privacy, and user experience in unprecedented ways.
Read More-